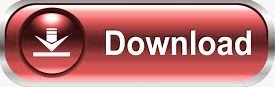

#IMAGEJ BATCH PROCESSING FREE#
It is not restricted by the resolution limitations and it is noise free but usually cannot imitate defects of the optical set-up, as well as the experimental one. The second approach is to calculate a theoretical PSF using one of the models approximating the optical set-up. This solution accounts for many of the deviations in the experimental set-up (such as flaws in the optical pathway), but the resolution of the PSF is limited by a signal-to-noise ratio, and it requires a demanding experimental procedure. First is to measure the actual PSF on a microscope using, for example, nanometre-sized fluorescent beads. The goal of deconvolution algorithms is to reverse this process. Each of the steps can be automated to some extent. The final step is the data extraction, statistical analysis (if applicable), and preparation of a figure for presentation. reconstructed or deconvolved) and then subjected to image analysis, or analysed without any additional intermediate steps. After acquisition, depending on a type of microscopy and analysis required, image may be enhanced (e.g. Panel C shows the microscopy image acquisition-analysis pipeline. The brightness and contrast parameter was chosen to cover 50–99% of the pixel brightness histogram, separately in the predeconvolution and postdeconvolution images. The image was collected as a z-stack of 21 slices, one of the representative intermediate slices is presented. Panel B shows an example of a deconvolved image of immunofluorescence staining of proteasomes (PSMA2 marker) in MDA-MB-231 cells (full description in a study by Baster et al. Image saturation level was set to 0.01 (high saturation). The green lines show the orientations of the cross-sections.
#IMAGEJ BATCH PROCESSING GENERATOR#
Panel A shows exemplary cross-section images of a point spread function (PSF) generated with PSF Generator using Gibson-Lanni algorithm. a cytoskeleton or an organelle) is a superposition of many single bright points convolved with the PSF : It is shaped due to physical limitation of light, physical limitations of imaging environment, and also defects and imperfections present in the imaging system. Īn image of a single bright point is visible as a characteristic diffraction-based pattern called point spread function (PSF) ( Figure 1A) which is specific for every optical set-up.
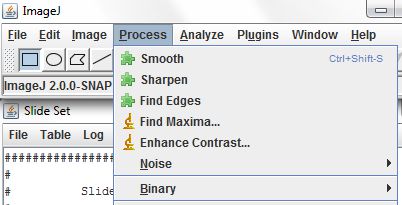
Secondly, in comparison to other techniques, the intensity-based quantitative analysis on deconvolved images is much more challenging due to nonlinear operations required for image reconstruction. Nevertheless, one of the main drawbacks of this technique is the requirement of vast amount of calculations, what significantly extends image preparation time. Based on a computational image reconstruction, it can improve both lateral and axial resolutions, allowing 3D imaging without any additional equipment cost. ĭeconvolution microscopy is one of a few techniques that do not require any additional hardware. There is a number of microscopy techniques allowing 3D imaging, such as confocal microscopy, 3D structured illumination microscopy, 3D stochastic optical reconstruction microscopy/photoactivated localization microscopy or light sheet microscopy, but most of them require additional, usually expensive, modules, which have to be mounted on a microscope. Unfortunately, it is much harder to reach high resolution along the Z optical axis, therefore three-dimensional (3D) imaging is much more challenging.
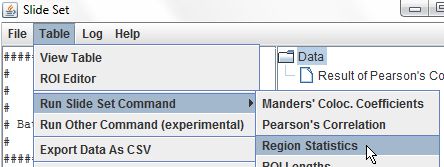
Using a relatively low-cost fluorescence microscope, it is possible to achieve the lateral resolution as low as 200 nm. The use of fluorescent probes allowed to tag specific proteins and structures within live or fixed cells and image them with high contrast and resolution. Fluorescence microscopy is one of the most commonly used techniques in life sciences.
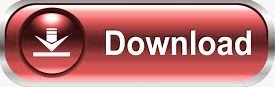